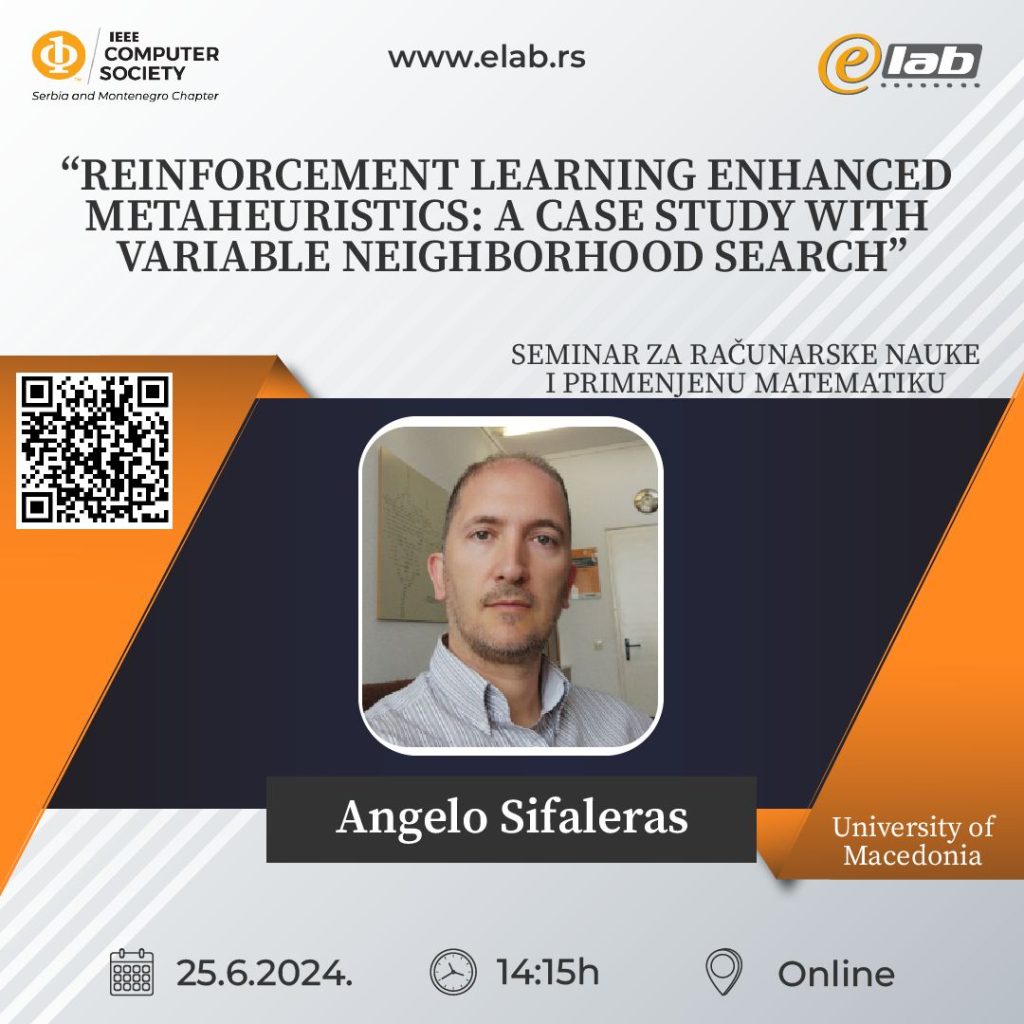
Datum i vreme: Utorak, 25.06.2024. u 14:15
Lokacija: Online
Predavač: Angelo Sifaleras, Department of Applied Informatics, University of Macedonia, Thessaloniki, Greece
Zajednički sastanak sa Seminarom za Veštačku inteligenciju.
Rezime: This presentation introduces a new hyperheuristic approach which combines Reinforcement Learning and Variable Neighborhood Search (VNS) and it is called Bandit VNS, in order to improve solutions for the Capacitated Vehicle Routing Problem (CVRP). The Multi-Armed Bandit framework, refined by the UCB algorithm, dynamically selects the most promising neighborhood structures, ad- dressing the challenge of identifying the optimal sequence of local search operators. Adaptive Windowing enhances the system’s responsiveness to the evolving optimization landscape. Our comprehensive analysis includes evaluating various UCB algorithm modifications and incorporating parallel computing paradigms that augment Bandit VNS. Empirical validations conducted on benchmark CVRP instances highlight a 25% enhancement in solution quality when compared to the conventional General Variable Neighborhood Search method using standard library instances of medium and large size. Our research sets a precedent for future explorations into AI-driven metaheuristics, promising advancements in optimization theory and practice.
This is a joint work by: Panagiotis Kalatzantonakis, Angelo Sifaleras, and Nikolaos Samaras, all from the Department of Applied Informatics, of the University of Macedonia, Thessaloniki, Greece. The talk will mainly summarize the findings of the paper: [Kalatzantonakis P., Sifaleras A., and Samaras N., “A reinforcement learning – variable neighborhood search method for the capacitated vehicle routing problem”, Expert Systems with Applications, Elsevier, Vol. 213, Article ID 118812, 2023.], but will also discuss about potential future work.
Rukovodioci seminara:
MI SANU: Vera Kovačević-Vujčić, Milan Dražić
FON: Zorica Bogdanović, Marijana
Despotović- Zrakić
IEEE: Božidar Radenković